New forces are going to cross the river by feeling Musk again
2024-07-17
Before venturing into the river by feeling for Musk, He Xiaopeng first felt around with a former colleague.
"Tesla's R&D progress is 1.5-2 years ahead of domestic intelligent driving." In early July, a statement by Gu Junli, former Vice President of XPeng's autonomous driving R&D, sparked controversy.
Since then, almost no car industry magnate dares to directly comment on the specific gap between Tesla and Chinese car companies in intelligent driving. Recently, when it was He Xiaopeng's turn to comment, he only lightly praised, saying that in the past, he always thought that Waymo, under Google's parent company, was a god-like existence, but after experiencing Tesla's FSD V12 version in San Francisco in June, he felt "very, very surprised, it's completely not the same version as before."
The secret weapon that surprised He Xiaopeng comes from Tesla's new version of end-to-end autonomous driving technology, FSD V12, which was officially launched at the beginning of this year.Tesla FSD V12, the most significant change is the adoption of an "end-to-end" architecture, which means data from sensors such as cameras is input at one end, and the other end directly outputs how the car should be driven.
Prior to this, autonomous driving relied on a modular approach, where perception, prediction, planning, and other functions were divided into separate modules. This approach was entirely dependent on engineers writing extensive code to establish driving rules, thereby instructing the car which rules to follow in specific situations.
Advertisement
If we liken the modular approach to Siri in an iPhone, then the advent of end-to-end is undoubtedly akin to the arrival of the ChatGPT era.
He Xiaopeng, who proclaimed that his company is one of only two globally and the only one in China to achieve mass production and deployment of end-to-end large models, expressed no concern about competition from Tesla when discussing the potential entry of Tesla FSD into China by the end of the year.
Similarly, Huawei, which does not want to lag behind Tesla, also began to emphasize "end-to-end" technology when it released the Qian Kun ADS 3.0 architecture in April. In July, during the Ideal Intelligent Driving Summer Conference, the official also unveiled a brand-new autonomous driving technology architecture based on end-to-end models, VLM visual language models, and world models. Recently, NIO also officially announced its intelligent driving technology architecture NADArch 2.0, formally introducing the end-to-end architecture of the world model.The concept of the "world model" as idealized by NIO, also somewhat carries the shadow of Tesla.
At the 2021 Tesla AI Day, through simulation, leveraging the supercomputing power of Dojo, Musk outlined a feasible path for Tesla towards full self-driving capability. This involves using virtual simulation technology to test edge cases and other unexpected issues that may be encountered during the driving process, "reproducing self-driving scenarios with self-driving technology," said Musk.
A decade ago, at the inception of "NIO, XPeng, and Li Auto," they all followed Tesla's lead in opening up consumer awareness of electric vehicles. Ten years later, facing the new round of technological revolution brought about by AI large models, the new forces are once again closely following Tesla's pace.
And this competition may be even more intense than it was a decade ago.
The year 2014 was a pivotal moment in the history of smart cars. From this year on, the paradigm shift in the automotive industry swept the globe, and the automotive industry entered an era of cross-disciplinary innovation: the traditional mechanical and manufacturing models were gradually surpassed by emerging new energy and electronic and electrical technologies.The advent of a new era where software drives cars marks the beginning of a paradigm shift in the automotive industry towards an economic model based on ICT and internet technologies. The marginal cost effect, which was originally applicable to the consumer electronics industry, begins to take effect in the automotive sector.
Despite the high initial costs of intelligent development, once successful, the marginal cost of replication and expansion becomes infinitely low. "Everyone has to compete desperately in R&D and technology; this is why in the consumer electronics industry, such as mobile phones, it's a winner-takes-all game," said Lei Jun in a recent exclusive interview with Li Xiang.
Now, the prospects and pressures of a winner-takes-all scenario have both come to the forefront for new forces.
A
Questioning Tesla, understanding Tesla, and then becoming a part of Tesla is a recurring scene in the automotive circle.When Tesla was developing the Autopilot feature for assisted self-driving in 2013, it dutifully followed the path pioneered by Google, which involved using sensors such as lidar and cameras to collect data. After manual annotation, engineers would then write driving rule code to determine how the car should operate.
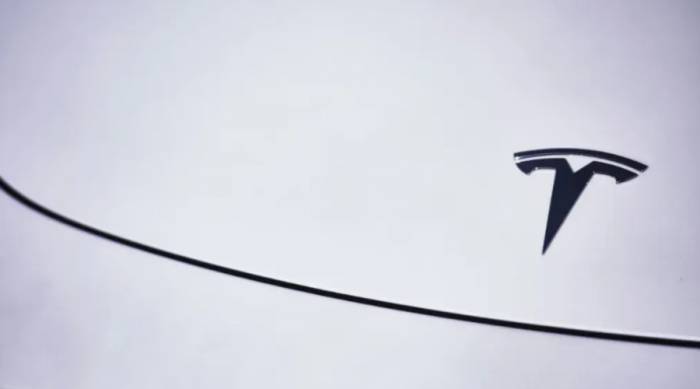
The shift occurred in 2021. In order to reduce costs and more rapidly popularize self-driving features, Musk decided to abandon lidar and high-precision maps in favor of a pure vision approach.
In May 2021, Tesla officially decided to remove the millimeter-wave radar that was previously standard on mass-produced vehicles, retaining only the eight cameras throughout the car to collect external environmental data for Tesla's Full Self-Driving (FSD).
On July 31, 2021, Tesla began rolling out the new version of FSD, FSD Beta V9.1, which was the first assisted driving suite to use the "Tesla Vision" approach.
Since then, the field of autonomous driving has been divided into two major schools of thought—those who advocate for a pure vision approach, led by Tesla, and those who support the use of lidar, led by companies such as Xiaopeng and NIO.One of the significant reasons that emboldened Musk to abandon lidar was Tesla's update of the BEV (Bird's Eye View) perception model based on the Transformer, which does not rely on high-precision maps to achieve assisted autonomous driving functions.
Beyond the BEV + Transformer architecture, Tesla subsequently integrated Occupancy Network technology (OCC), further enhancing the navigation accuracy of the pure vision solution.
A year after Tesla switched to a pure vision solution, in the second half of 2022, companies like NIO, Xpeng, Huawei, and BYD began to follow Tesla's lead, turning towards the development of BEV + Transformer solutions that do not depend on high-precision maps.
Han Bing, the head of BYD's intelligent driving business, even stated at an event in the middle of last year: "BEV is the opportunity for BYD to overtake in high-level intelligent driving."
It is precisely because of the elimination of the constraints of high-precision maps that in 2023, Chinese car manufacturers have launched a new competition to compare the speed of launching NOA (Navigation on Autopilot) in cities.Yu Chengdong once lamented that Huawei had invested in Shanghai for a year or two without completing the collection of urban high-precision maps, "Even a small road in Shanghai takes a long time to deal with."
In addition to accelerating the pace of NOA city deployment, the pure vision solution led by Tesla has also shown new forces the possibility of bringing high-level intelligent driving technology to affordable models.
The Xiaomi SU7, released in March, uses a lidar solution for its Pro/Max versions, while the standard version adopts a pure vision solution; the Xiaopeng M03, which will be launched in August, will also be equipped with different intelligent driving solutions, marking Xiaopeng's first application of the pure vision approach in mass-produced vehicles; NIO's second brand, Ledao L60, which will be launched in September, has also canceled the lidar out of cost considerations and switched to a pure vision solution.
In order to capture more cooperation orders from car manufacturers, Huawei officially released its vision-based intelligent driving solution this year - HUAWEI ADS Basic Edition, which claims to achieve intelligent assisted driving on national highways and urban expressways, as well as intelligent parking functions, and has been successively applied to the Smart S7 and Deep Blue S07.
With the explosion of AI large models in 2022, the end-to-end intelligent driving new route ignited by Tesla has become the new goal for car companies to compete after the "BEV + Transformer + OCC occupancy network" intelligent driving solution.To achieve end-to-end capabilities, automotive companies' visual neural networks require the collection of a larger volume, more diverse, and more realistic data samples, while utilizing data with lower latency computing power for analysis.
This directly led to the birth of Tesla's supercomputing system, Dojo, three years ago.
At the 2021 Tesla AI Day, Musk claimed that Dojo's efficiency had surpassed that of NVIDIA GPUs and Google TPUs. In pursuit of the goal of being the strongest on the planet, Tesla independently developed its first AI training chip, the D1. The D1, with a 7nm process, has a computing power of 362 TFLOPS (equivalent to 362 trillion floating-point operations per second).
Before the D1, Musk had already secured Tesla's position as the world's first automaker to develop its own intelligent driving chip with the birth of the FSD inference chip.After Tesla began mass-producing the FSD chip in 2019, NIO, XPeng, and Li Auto successively established their own chip teams in 2020.
In the field of smart automobiles, in order to achieve better performance optimization, integrated in-house development from chips to algorithms and operating systems has become the direction that more and more car manufacturers are striving to conquer. This is particularly evident among leading new energy vehicle companies. He Xiaopeng has also publicly expressed his view that "a controllable model of integrated hardware and software, like Apple's, will appear more and more in smart car enterprises."
As Tesla goes further down the path of integrated hardware and software, the new forces are also stepping up their efforts to catch up.
Entering July 2024, after four years of chip development, NIO, XPeng, and Li Auto have finally achieved results: NIO announced at NIO IN 2024 that the world's first 5nm smart driving chip, the Shenxian NX9031, has been successfully taped out. This chip is expected to be first equipped in NIO's flagship sedan ET9 in the first quarter of 2025.At the same time, according to a scoop from 36Kr Auto, the intelligent driving chip developed by Xiaopeng Motors has also been sent for tape-out, "with an expected return in August."
Li Auto's intelligent driving chip is also expected to be taped out within this year.
Better cost control has become one of the main reasons for the new forces to start making their own chips.
"If Li Auto develops its own inference chip, it can achieve a cost similar to that of Tesla, because the algorithm is in its own hands, including the entire training platform and training chip that it will develop later," Li Xiang once said, and even evaluated the specific cost reduction of Tesla's AI training chip D1, "It can probably achieve one-sixth of the cost of Nvidia's A100."
It is worth mentioning that under the crisis of Nvidia's chip supply disruption, the self-development of chips by the new forces has also begun to take on the strategic value of safeguarding the security of the supply chain.Moreover, as chips continue to iterate, the cost optimization space they bring to car manufacturers will further expand. At the second-quarter financial report meeting, Musk stated that the next-generation Tesla AI chip, known as HW5, "from the perspective of inference capability, it can be compared to NVIDIA's GB 200."
However, whether it is pushing for end-to-end solutions or developing their own chips, for most new forces still in the loss-making stage, it will be a pressured investment.
High-computing power chips for intelligent driving have long development cycles, require substantial financial investment, and come with high uncertainty. Statistical data shows that the research and mass production of a high-computing power chip will cost at least several billion yuan.
Yu Kai, the founder and CEO of Horizon Robotics, which supplies intelligent driving chips to car manufacturers, even bluntly stated that car manufacturers should carefully consider the issue of developing their own intelligent driving chips. "Developing in-house and using external resources are strategic choices. If a car factory's annual sales expectation is less than 1 million vehicles, the overall efficiency of the funds actually does not make financial sense."
This tests the economies of scale of car manufacturers. Only by selling more vehicles can they spread these R&D expenditures and leverage the cost advantages of developing their own chips.Looking at the global new energy vehicle manufacturers, in 2023, only BYD and Tesla have achieved the goal of selling one million vehicles annually.
It is even more undeniable that economies of scale will also help automakers gain more data support in end-to-end competition.
The upper limit of the end-to-end model is very high, with the potential to reach the L5 level of autonomous driving, but its lower limit is very low. In some basic conditions, it may not even be comparable to modular solutions. For example, after Tesla's FSD V12 version, which adopts an end-to-end approach, was launched, many people tested the vehicle and found low-level errors such as the car hitting the curb and damaging the wheel hub.
The main method to avoid the aforementioned low-level errors is to feed more resources to the end-to-end model, including computing power, algorithms, and data.At last year's financial report meeting, Musk discussed the importance of data for autonomous driving models: "Training with 1 million video clips is barely sufficient; 2 million is a bit better; 3 million, and you'll feel Wow; by the time you reach 10 million, it becomes incredible."
However, not all driving data can be used to train end-to-end models. In this regard, cars, like large models like ChatGPT, are tested by the manufacturer's attention to data details.
"In the new era of data-centric AI, whether a model can stand out actually depends mainly on the data," Li Zhen, a practitioner of large models, once explained to Wuji Caijing (ID: wujicaijing). The many devilish details hidden in the data can even determine the success or failure of large model products.
Google is a cautionary tale. In terms of computing power and algorithms, Google is not inferior to OpenAI, and may even be stronger, but it was precisely by leveraging data training based on human feedback that OpenAI managed to create ChatGPT ahead of Google.
"Why is Tesla so far ahead? It's because it has sales as a foundation," Gu Junli believes that to catch up with Tesla in business models, it is necessary to achieve product scale.The window for new forces to catch up with Tesla is narrowing. According to Musk's prediction, with the release of the FSD V12.5 or 12.6 version, as early as the end of this year, countries and regions such as China and Europe could receive approval for deployment.
Beyond economies of scale, Musk has also set an entry threshold for this end-to-end competition with Tesla's self-sustaining capabilities. On the day of his visit to China in April, Musk posted, "Tesla will invest about $10 billion this year in AI training and inference, the latter mainly for cars. Any company that does not reach such a level of expenditure and cannot efficiently accomplish this task cannot compete."
After enduring the challenge of mass production over a decade, a new round of elimination is once again facing the new forces.
Comments